Introduction to Sentiment Analysis in Python
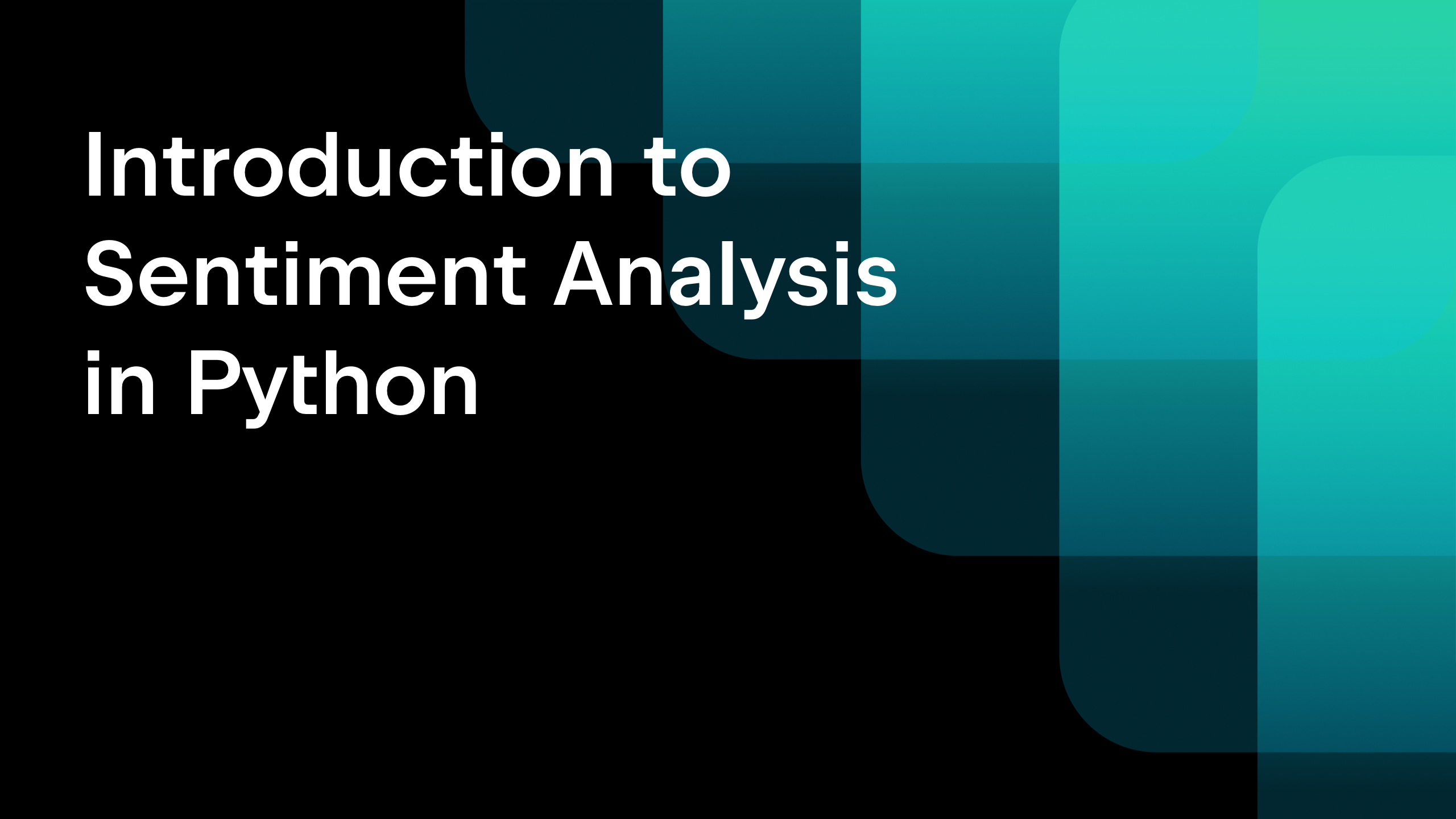
Sentiment analysis is one of the most popular ways to analyze text. It allows us to see at a glance how people are feeling across a wide range of areas and has useful applications in fields like customer service, market and product research, and competitive analysis.
Like any area of natural language processing (NLP), sentiment analysis can get complex. Luckily, Python has excellent packages and tools that make this branch of NLP much more approachable.
In this blog post, we’ll explore some of the most popular packages for analyzing sentiment in Python, how they work, and how you can train your own sentiment analysis model using state-of-the-art techniques. We’ll also look at some PyCharm features that make working with these packages easier and faster.
What is sentiment analysis?
Sentiment analysis is the process of analyzing a piece of text to determine its emotional tone. As you can probably see from this definition, sentiment analysis is a very broad field that incorporates a wide variety of methods within the field of natural language processing.
There are many ways to define “emotional tone”. The most commonly used methods determine the valence or polarity of a piece of text – that is, how positive or negative the sentiment expressed in a text is. Emotional tone is also usually treated as a text classification problem, where text is categorized as either positive or negative.
Take the following Amazon product review:
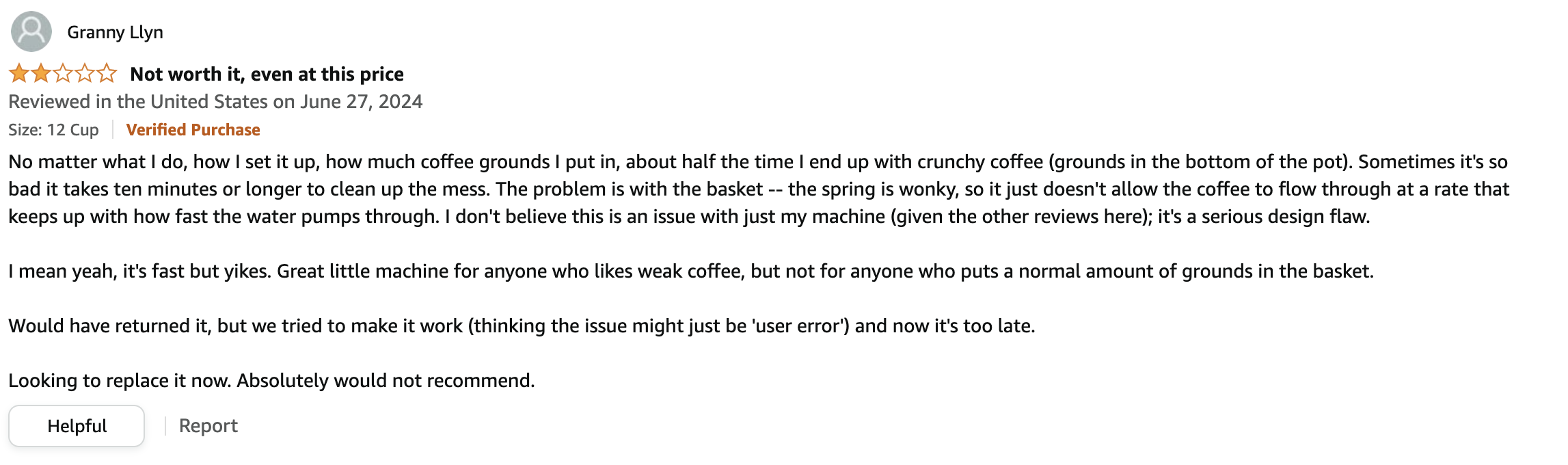
This is obviously not a happy customer, and sentiment analysis techniques would classify this review as negative.
Contrast this with a much more satisfied buyer:
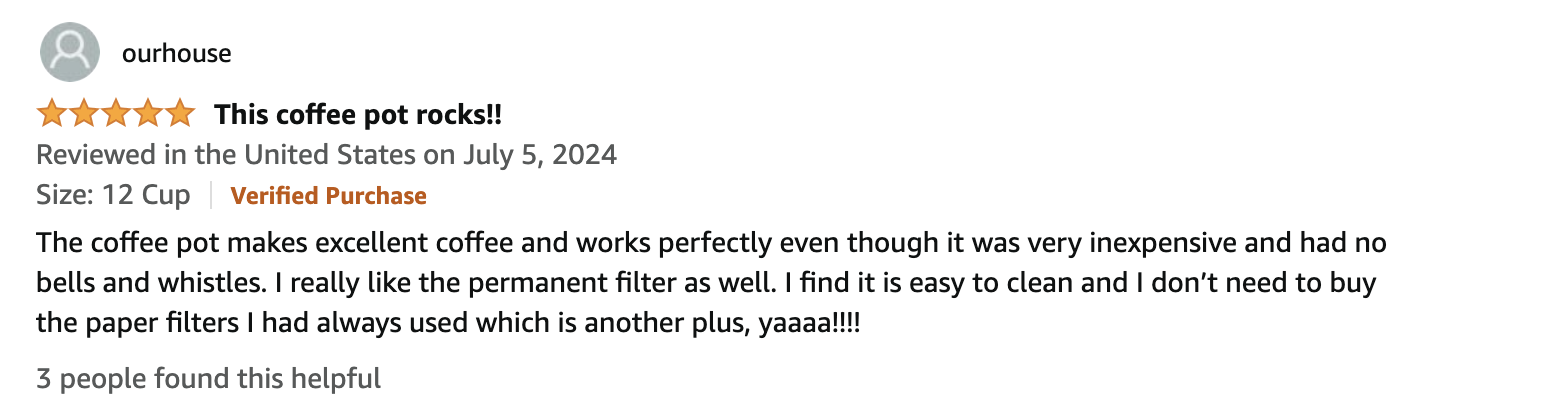
This time, sentiment analysis techniques would classify this as positive.
Different types of sentiment analysis
There are multiple ways of extracting emotional information from text. Let’s review a few of the most important ones.
Ways of defining sentiment
First, sentiment analysis approaches have several different ways of defining sentiment or emotion.
Binary: This is where the valence of a document is divided into two categories, either positive or negative, as with the SST-2 dataset. Related to this are classifications of valence that add a neutral class (where a text expresses no sentiment about a topic) or even a conflict class (where a text expresses both positive and negative sentiment about a topic).
Some sentiment analyzers use a related measure to classify texts into subjective or objective.
Fine-grained: This term describes several different ways of approaching sentiment analysis, but here it refers to breaking down positive and negative valence into a Likert scale. A well-known example of this is the SST-5 dataset, which uses a five-point Likert scale with the classes very positive, positive, neutral, negative, and very negative.
Continuous: The valence of a piece of text can also be measured continuously, with scores indicating how positive or negative the sentiment of the writer was. For example, the VADER sentiment analyzer gives a piece of text a score between –1 (strongly negative) and 1 (strongly positive), with scores close to 0 indicating a neutral sentiment.
Emotion-based: Also known as emotion detection or emotion identification, this approach attempts to detect the specific emotion being expressed in a piece of text. You can approach this in two ways. Categorical emotion detection tries to classify the sentiment expressed by a text into one of a handful of discrete emotions, usually based on the Ekman model, which includes anger, disgust, fear, joy, sadness, and surprise. A number of datasets exist for this type of emotion detection. Dimensional emotional detection is less commonly used in sentiment analysis and instead tries to measure three emotional aspects of a piece of text: polarity, arousal (how exciting a feeling is), and dominance (how restricted the emotional expression is).
Levels of analysis
We can also consider different levels at which we can analyze a piece of text. To understand this better, let’s consider another review of the coffee maker:
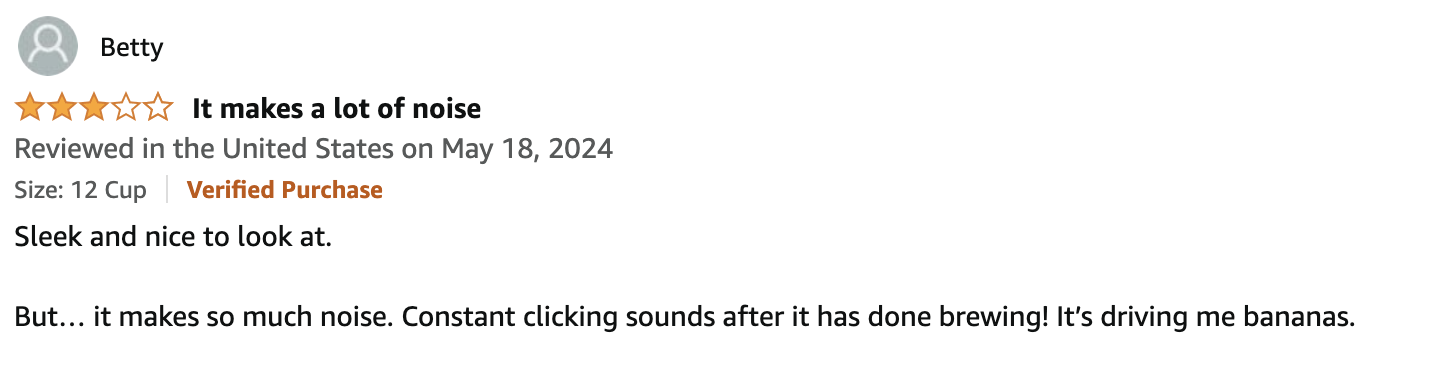
Document-level: This is the most basic level of analysis, where one sentiment for an entire piece of text will be returned. Document-level analysis might be fine for very short pieces of text, such as Tweets, but can give misleading answers if there is any mixed sentiment. For example, if we based the sentiment analysis for this review on the whole document, it would likely be classified as neutral or conflict, as we have two opposing sentiments about the same coffee machine.
Sentence-level: This is where the sentiment for each sentence is predicted separately. For the coffee machine review, sentence-level analysis would tell us that the reviewer felt positively about some parts of the product but negatively about others. However, this analysis doesn’t tell us what things the reviewer liked and disliked about the coffee machine.
Aspect-based: This type of sentiment analysis dives deeper into a piece of text and tries to understand the sentiment of users about specific aspects. For our review of the coffee maker, the reviewer mentioned two aspects: appearance and noise. By extracting these aspects, we have more information about what the user specifically did and did not like. They had a positive sentiment about the machine’s appearance but a negative sentiment about the noise it made.
Coupling sentiment analysis with other NLP techniques
Intent-based: In this final type of sentiment analysis, the text is classified in two ways: in terms of the sentiment being expressed, and the topic of the text. For example, if a telecommunication company receives a ticket complaining about how often their service goes down, they could classify the text intent or topic as service reliability and the sentiment as negative. As with aspect-based sentiment analysis, this analysis gives the company much more information than knowing whether their customers are generally happy or unhappy.
Applications of sentiment analysis
By now, you can probably already think of some potential use cases for sentiment analysis. Basically, it can be used anywhere that you could get text feedback or opinions about a topic. Organizations or individuals can use sentiment analysis to do social media monitoring and see how people feel about a brand, government entity, or topic.
Customer feedback analysis can be used to find out the sentiments expressed in feedback or tickets. Product reviews can be analyzed to see how satisfied or dissatisfied people are with a company’s products. Finally, sentiment analysis can be a key component in market research and competitive analysis, where how people feel about emerging trends, features, and competitors can help guide a company’s strategies.
How does sentiment analysis work?
At a general level, sentiment analysis operates by linking words (or, in more sophisticated models, the overall tone of a text) to an emotion. The most common approaches to sentiment analysis fall into one of the three methods below.
Lexicon-based approaches
These methods rely on a lexicon that includes sentiment scores for a range of words. They combine these scores using a set of rules to get the overall sentiment for a piece of text. These methods tend to be very fast and also have the advantage of yielding more fine-grained continuous sentiment scores. However, as the lexicons need to be handcrafted, they can be time-consuming and expensive to produce.
Machine learning models
These methods train a machine learning model, most commonly a Naive Bayes classifier, on a dataset that contains text and their sentiment labels, such as movie reviews. In this model, texts are generally classified as positive, negative, and sometimes neutral. These models also tend to be very fast, but as they usually don’t take into account the relationship between words in the input, they may struggle with more complex texts that involve qualifiers and negations.
Large language models
These methods rely on fine-tuning a pre-trained transformer-based large language model on the same datasets used to train the machine learning classifiers mentioned earlier. These sophisticated models are capable of modeling complex relationships between words in a piece of text but tend to be slower than the other two methods.
Sentiment analysis in Python
Python has a rich ecosystem of packages for NLP, meaning you are spoiled for choice when doing sentiment analysis in this language.
Let’s review some of the most popular Python packages for sentiment analysis.
The best Python libraries for sentiment analysis
VADER
VADER (Valence Aware Dictionary and Sentiment Reasoner) is a popular lexicon-based sentiment analyzer. Built into the powerful NLTK package, this analyzer returns four sentiment scores: the degree to which the text was positive, neutral, or negative, as well as a compound sentiment score. The positive, neutral, and negative scores range from 0 to 1 and indicate the proportion of the text that was positive, neutral, or negative. The compound score ranges from –1 (extremely negative) to 1 (extremely positive) and indicates the overall sentiment valence of the text.
Let’s look at a basic example of how it works:
from nltk.sentiment.vader import SentimentIntensityAnalyzer import nltk
We first need to download the VADER lexicon.
nltk.download('vader_lexicon')
We can then instantiate the VADER SentimentIntensityAnalyzer()
and extract the sentiment scores using the polarity_scores()
method.
analyzer = SentimentIntensityAnalyzer() sentence = "I love PyCharm! It's my favorite Python IDE." sentiment_scores = analyzer.polarity_scores(sentence) print(sentiment_scores)
{'neg': 0.0, 'neu': 0.572, 'pos': 0.428, 'compound': 0.6696}
We can see that VADER has given this piece of text an overall sentiment score of 0.67 and classified its contents as 43% positive, 57% neutral, and 0% negative.
VADER works by looking up the sentiment scores for each word in its lexicon and combining them using a nuanced set of rules. For example, qualifiers can increase or decrease the intensity of a word’s sentiment, so a qualifier such as “a bit” before a word would decrease the sentiment intensity, but “extremely” would amplify it.
VADER’s lexicon includes abbreviations such as “smh” (shaking my head) and emojis, making it particularly suitable for social media text. VADER’s main limitation is that it doesn’t work for languages other than English, but you can use projects such as vader-multi
as an alternative. I wrote about how VADER works if you’re interested in taking a deeper dive into this package.
NLTK
Additionally, you can use NLTK to train your own machine learning-based sentiment classifier, using classifiers from scikit-learn
.
There are many ways of processing the text to feed into these models, but the simplest way is doing it based on the words that are present in the text, a type of text modeling called the bag-of-words approach. The most straightforward type of bag-of-words modeling is binary vectorisation, where each word is treated as a feature, with the value of that feature being either 0 or 1 (whether the word is absent or present in the text, respectively).
If you’re new to working with text data and NLP, and you’d like more information about how text can be converted into inputs for machine learning models, I gave a talk on this topic that provides a gentle introduction.
You can see an example in the NLTK documentation, where a Naive Bayes classifier is trained to predict whether a piece of text is subjective or objective. In this example, they add an additional negation qualifier to some of the terms based on rules which indicate whether that word or character is likely involved in negating a sentiment expressed elsewhere in the text. Real Python also has a sentiment analysis tutorial on training your own classifiers using NLTK, if you want to learn more about this topic.
Pattern and TextBlob
The Pattern package provides another lexicon-based approach to analyzing sentiment. It uses the SentiWordNet lexicon, where each synonym group (synset) from WordNet is assigned a score for positivity, negativity, and objectivity. The positive and negative scores for each word are combined using a series of rules to give a final polarity score. Similarly, the objectivity score for each word is combined to give a final subjectivity score.
As WordNet contains part-of-speech information, the rules can take into account whether adjectives or adverbs preceding a word modify its sentiment. The ruleset also considers negations, exclamation marks, and emojis, and even includes some rules to handle idioms and sarcasm.
However, Pattern as a standalone library is only compatible with Python 3.6. As such, the most common way to use Pattern is through TextBlob. By default, the TextBlob sentiment analyzer uses its own implementation of the Pattern library to generate sentiment scores.
Let’s have a look at this in action:
from textblob import TextBlob
You can see that we run the TextBlob method over our text, and then extract the sentiment using the sentiment
attribute.
pattern_blob = TextBlob("I love PyCharm! It's my favorite Python IDE.") sentiment = pattern_blob.sentiment print(f"Polarity: {sentiment.polarity}") print(f"Subjectivity: {sentiment.subjectivity}")
Polarity: 0.625 Subjectivity: 0.6
For our example sentence, Pattern in TextBlob gives us a polarity score of 0.625 (relatively close to the score given by VADER), and a subjectivity score of 0.6.
But there’s also a second way of getting sentiment scores in TextBlob. This package also includes a pre-trained Naive Bayes classifier, which will label a piece of text as either positive or negative, and give you the probability of the text being either positive or negative.
To use this method, we first need to download both the punkt
module and the movie-reviews
dataset from NLTK, which is used to train this model.
import nltk nltk.download('movie_reviews') nltk.download('punkt') from textblob import TextBlob from textblob.sentiments import NaiveBayesAnalyzer
Once again, we need to run TextBlob
over our text, but this time we add the argument analyzer=NaiveBayesAnalyzer()
. Then, as before, we use the sentiment attribute to extract the sentiment scores.
nb_blob = TextBlob("I love PyCharm! It's my favorite Python IDE.", analyzer=NaiveBayesAnalyzer()) sentiment = nb_blob.sentiment print(sentiment)
Sentiment(classification='pos', p_pos=0.5851800554016624, p_neg=0.4148199445983381)
This time we end up with a label of pos
(positive), with the model predicting that the text has a 59% probability of being positive and a 41% probability of being negative.
spaCy
Another option is to use spaCy for sentiment analysis. spaCy is another popular package for NLP in Python, and has a wide range of options for processing text.
The first method is by using the spacytextblob plugin to use the TextBlob sentiment analyzer as part of your spaCy pipeline. Before you can do this, you’ll first need to install both spacy
and spacytextblob
and download the appropriate language model.
import spacy import spacy.cli from spacytextblob.spacytextblob import SpacyTextBlob spacy.cli.download("en_core_web_sm")
We then load in this language model and add spacytextblob
to our text processing pipeline. TextBlob can be used through spaCy’s pipe
method, which means we can include it as part of a more complex text processing pipeline, including preprocessing steps such as part-of-speech tagging, lemmatization, and named-entity recognition. Preprocessing can normalize and enrich text, helping downstream models to get the most information out of the text inputs.
nlp = spacy.load('en_core_web_sm') nlp.add_pipe('spacytextblob')
For now, we’ll just analyze our sample sentence without preprocessing:
doc = nlp("I love PyCharm! It's my favorite Python IDE.") print('Polarity: ', doc._.polarity) print('Subjectivity: ', doc._.subjectivity)
Polarity: 0.625 Subjectivity: 0.6
We get the same results as when using TextBlob above.
A second way we can do sentiment analysis in spaCy is by training our own model using the TextCategorizer class. This allows you to train a range of spaCY created models using a sentiment analysis training set. Again, as this can be used as part of the spaCy pipeline, you have many options for pre-processing your text before training your model.
Finally, you can use large language models to do sentiment analysis through spacy-llm. This allows you to prompt a variety of proprietary large language models (LLMs) from OpenAI, Anthropic, Cohere, and Google to perform sentiment analysis over your texts.
This approach works slightly differently from the other methods we’ve discussed. Instead of training the model, we can use generalist models like GPT-4 to predict the sentiment of a text. You can do this either through zero-shot learning (where a prompt but no examples are passed to the model) or few-shot learning (where a prompt and a number of examples are passed to the model).
Transformers
The final Python package for sentiment analysis we’ll discuss is Transformers from Hugging Face.
Hugging Face hosts all major open-source LLMs for free use (among other models, including computer vision and audio models), and provides a platform for training, deploying, and sharing these models. Its Transformers package offers a wide range of functionality (including sentiment analysis) for working with the LLMs hosted by Hugging Face.
Understanding the results of sentiment analyzers
Now that we’ve covered all of the ways you can do sentiment analysis in Python, you might be wondering, “How can I apply this to my own data?”
To understand this, let’s use PyCharm to compare two packages, VADER and TextBlob. Their multiple sentiment scores offer us a few different perspectives on our data. We’ll use these packages to analyze the Amazon reviews dataset.
PyCharm Professional is a powerful Python IDE for data science that supports advanced Python code completion, inspections and debugging, rich databases, Jupyter, Git, Conda, and more – all out of the box. In addition to these, you’ll also get incredibly useful features like our DataFrame Column Statistics and Chart View, as well as Hugging Face integrations that make working with LLMs much quicker and easier. In this blog post, we’ll explore PyCharm’s advanced features for working with dataframes, which will allow us to get a quick overview of how our sentiment scores are distributed between the two packages.
If you’re now ready to get started on your own sentiment analysis project, you can activate your free three-month subscription to PyCharm. Click on the link below, and enter this promo code: PCSA24. You’ll then receive an activation code via email.
The first thing we need to do is load in the data. We can use the load_dataset()
method from the Datasets package to download this data from the Hugging Face Hub.
from datasets import load_dataset amazon = load_dataset("fancyzhx/amazon_polarity")
You can hover over the name of the dataset to see the Hugging Face dataset card right inside PyCharm, providing you with a convenient way to get information about Hugging Face assets without leaving the IDE.
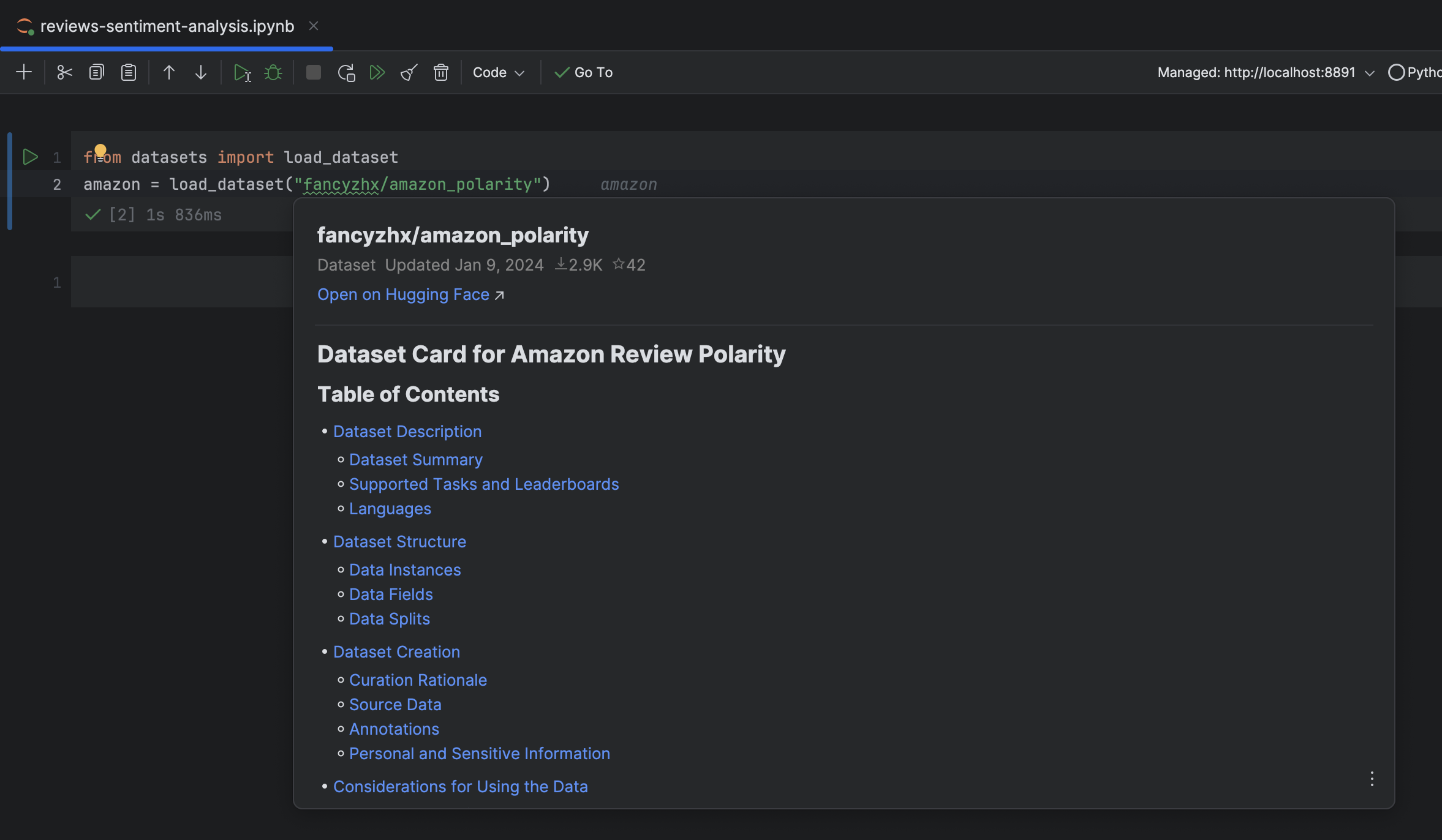
We can see the contents of this dataset here:
amazon
DatasetDict({ train: Dataset({ features: ['label', 'title', 'content'], num_rows: 3600000 }) test: Dataset({ features: ['label', 'title', 'content'], num_rows: 400000 }) })
The training dataset has 3.6 million observations, and the test dataset contains 400,000. We’ll be working with the training dataset in this tutorial.
We’ll now load in the VADER SentimentIntensityAnalyzer
and the TextBlob method.
from nltk.sentiment.vader import SentimentIntensityAnalyzer import nltk nltk.download("vader_lexicon") analyzer = SentimentIntensityAnalyzer()
from textblob import TextBlob
The training dataset has too many observations to comfortably visualize, so we’ll take a random sample of 1,000 reviews to represent the general sentiment of all the reviewers.
from random import sample sample_reviews = sample(amazon["train"]["content"], 1000)
Let’s now get the VADER and TextBlob scores for each of these reviews. We’ll loop over each review text, run them through the sentiment analyzers, and then attach the scores to a dedicated list.
vader_neg = [] vader_neu = [] vader_pos = [] vader_compound = [] textblob_polarity = [] textblob_subjectivity = [] for review in sample_reviews: vader_sent = analyzer.polarity_scores(review) vader_neg += [vader_sent["neg"]] vader_neu += [vader_sent["neu"]] vader_pos += [vader_sent["pos"]] vader_compound += [vader_sent["compound"]] textblob_sent = TextBlob(review).sentiment textblob_polarity += [textblob_sent.polarity] textblob_subjectivity += [textblob_sent.subjectivity]
We’ll then pop each of these lists into a pandas DataFrame as a separate column:
import pandas as pd sent_scores = pd.DataFrame({ "vader_neg": vader_neg, "vader_neu": vader_neu, "vader_pos": vader_pos, "vader_compound": vader_compound, "textblob_polarity": textblob_polarity, "textblob_subjectivity": textblob_subjectivity })
Now, we’re ready to start exploring our results.
Typically, this would be the point where we’d start creating a bunch of code for exploratory data analysis. This might be done using pandas’ describe
method to get summary statistics over our columns, and writing Matplotlib or seaborn code to visualize our results. However, PyCharm has some features to speed this whole thing up.
Let’s go ahead and print our DataFrame.
sent_scores
We can see a button in the top right-hand corner, called Show Column Statistics. Clicking this gives us two different options: Compact and Detailed. Let’s select Detailed.
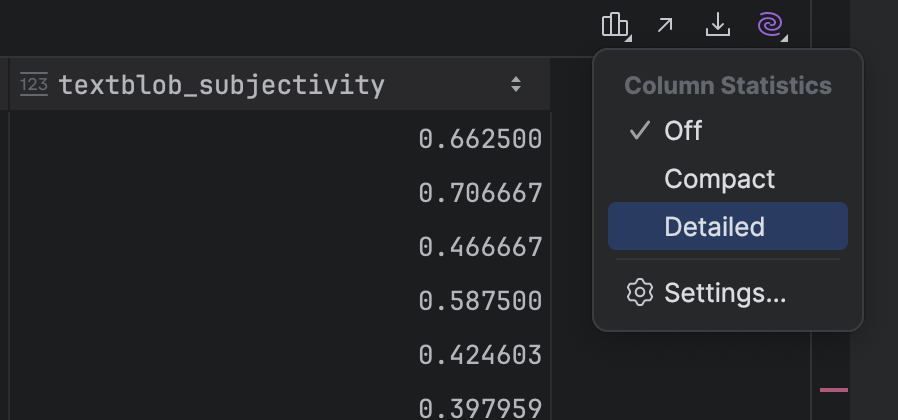
Now we have summary statistics provided as part of our column headers! Looking at these, we can see the VADER compound score has a mean of 0.4 (median = 0.6), while the TextBlob polarity score provides a mean of 0.2 (median = 0.2).
This result indicates that, on average, VADER tends to estimate the same set of reviews more positively than TextBlob does. It also shows that for both sentiment analyzers, we likely have more positive reviews than negative ones – we can dive into this in more detail by checking some visualizations.
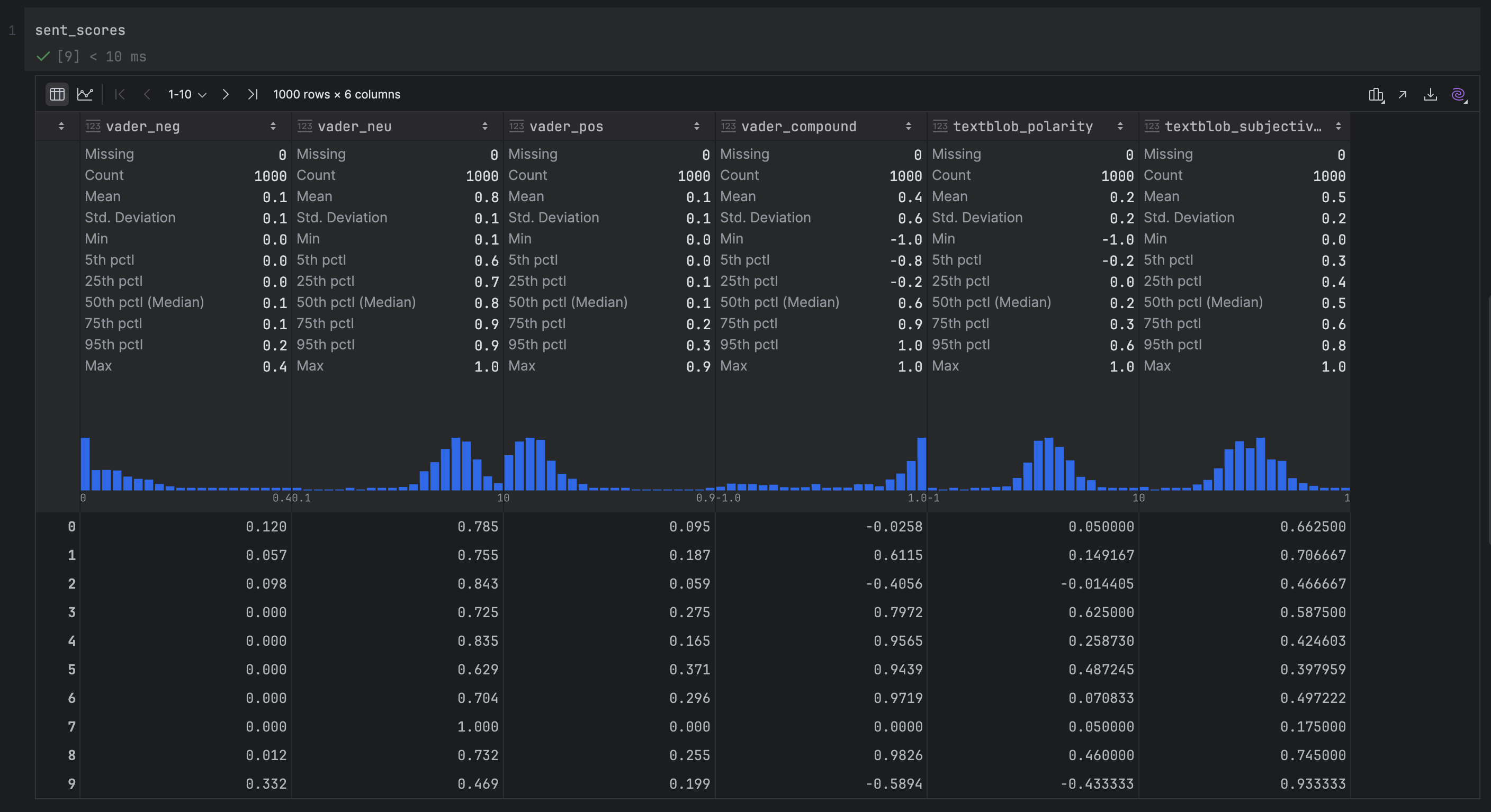
Another PyCharm feature we can use is the DataFrame Chart View. The button for this function is in the top left-hand corner.
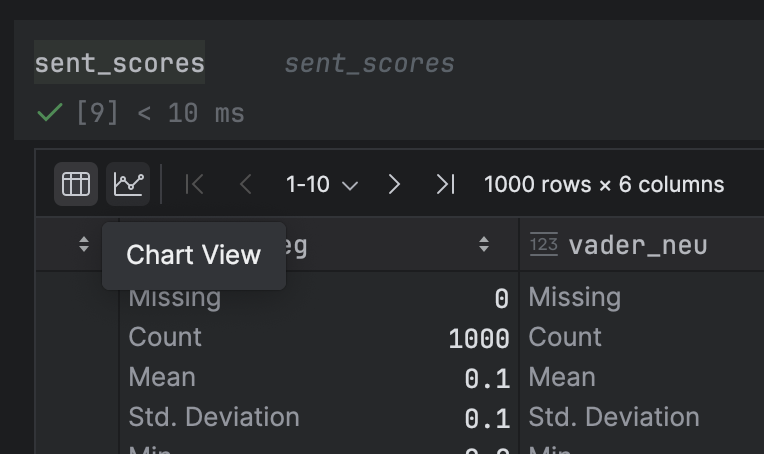
When we click on the button, we switch over to the chart editor. From here, we can create no-code visualizations straight from our DataFrame.
Let’s start with VADER’s compound score. To start creating this chart, go to Show Series Settings in the top right-hand corner.
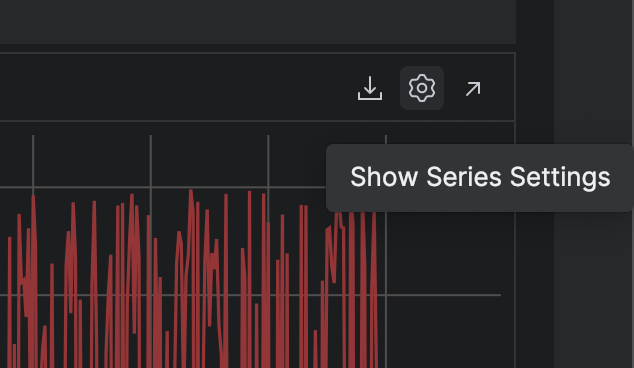
Remove the default values for X Axis and Y Axis. Replace the X Axis value with vader_compound
, and the Y Axis value with vader_compound
. Click on the arrow next to the variable name in the Y Axis field, and select count
.
Finally, select Histogram from the chart icons, just under Series Settings. We likely have a bimodal distribution for the VADER compound score, with a slight peak around –0.8 and a much larger one around 0.9. This peak likely represents the split of negative and positive reviews. There are also far more positive reviews than negative.
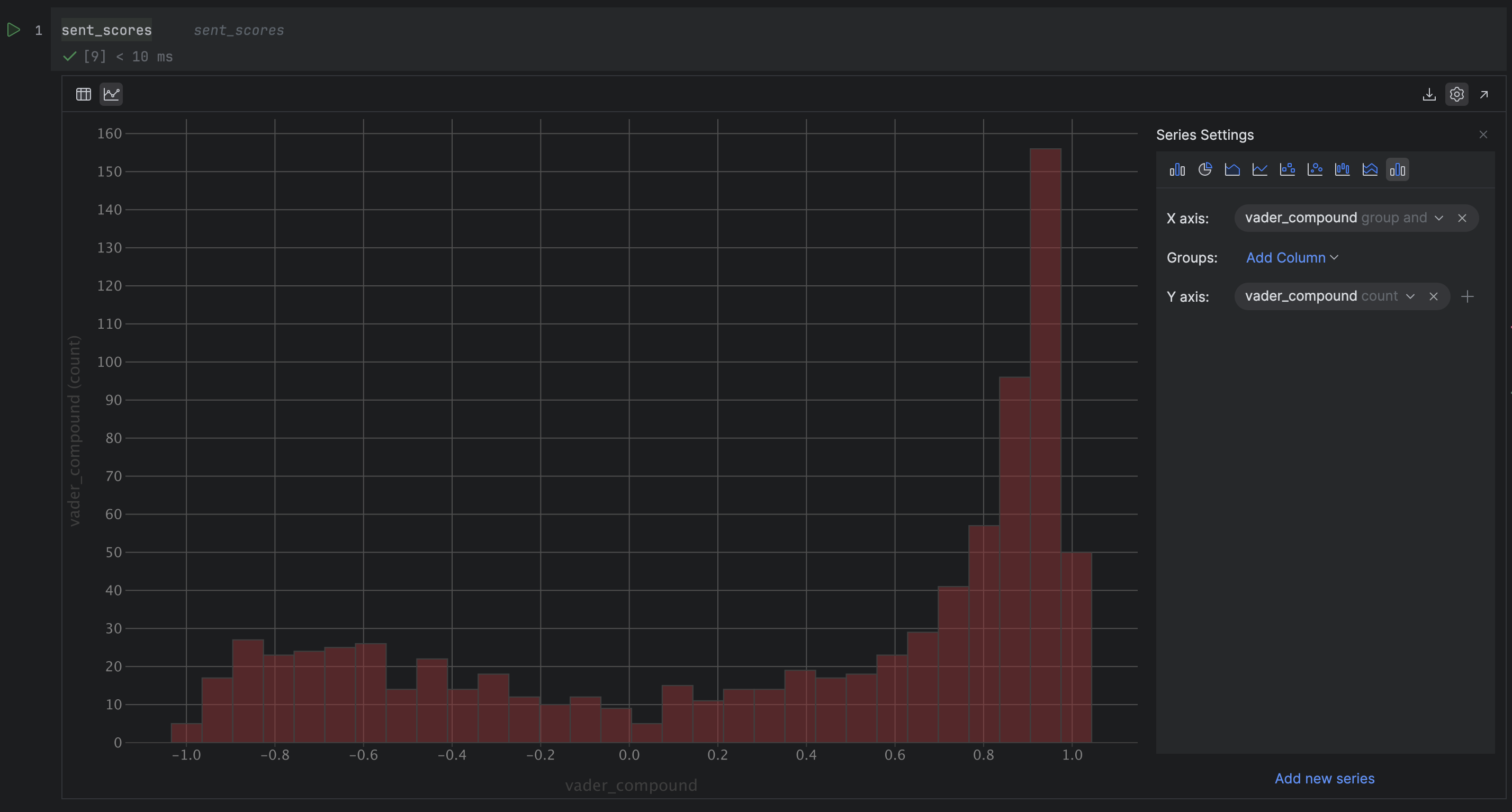
Let’s repeat the same exercise and create a histogram to see the distribution of the TextBlob polarity scores.
In contrast, TextBlob tends to rate most reviews as neutral, with very few reviews being strongly positive or negative. To understand why we have a discrepancy in the scores these two sentiment analyzers provide, let’s look at a review VADER rated as strongly positive and another that VADER rated strongly negative but that TextBlob rated as neutral.
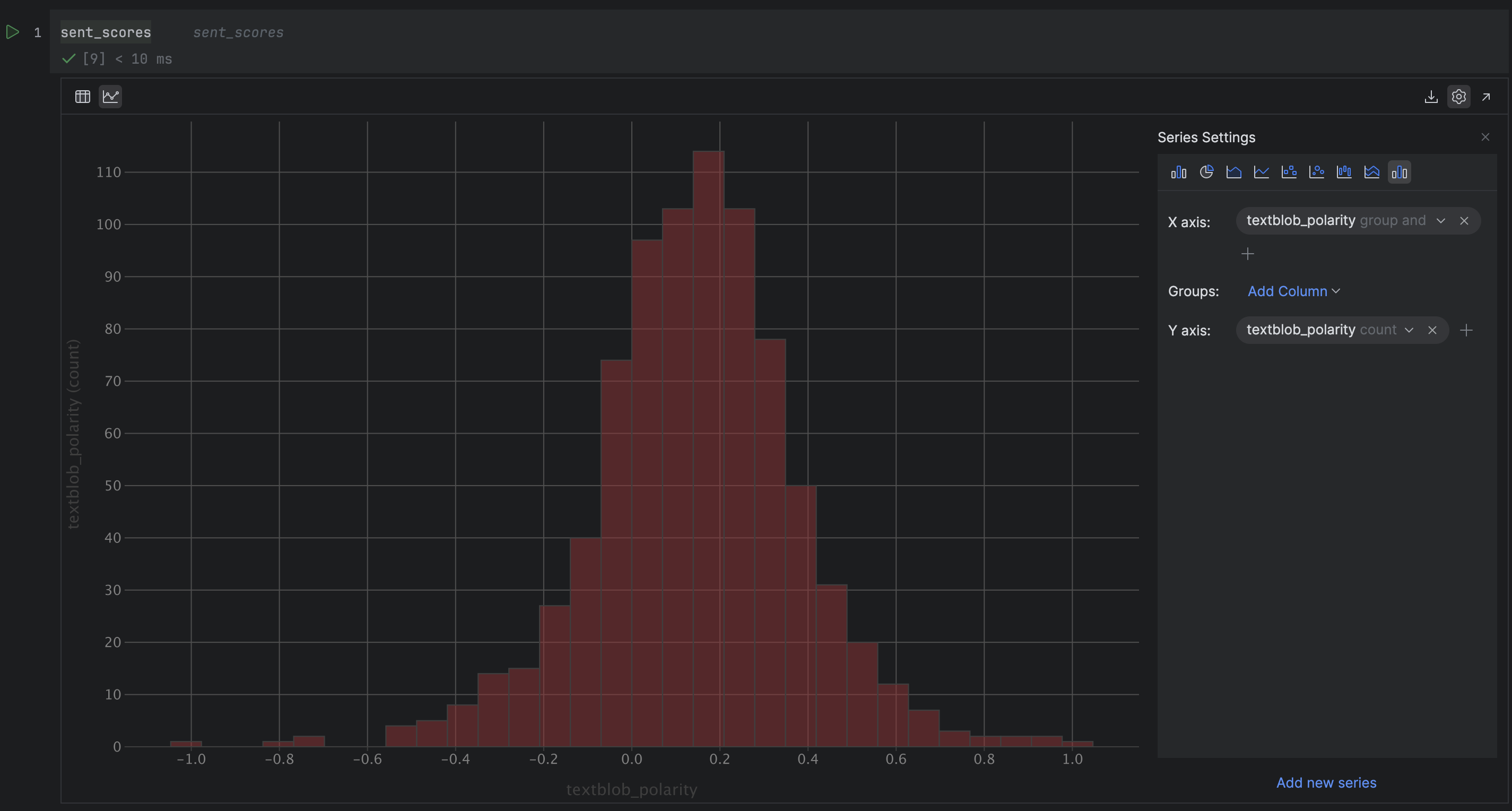
We’ll get the index of the first review where VADER rated them as positive but TextBlob rated them as neutral:
sent_scores[(sent_scores["vader_compound"] >= 0.8) & (sent_scores["textblob_polarity"].between(-0.1, 0.1))].index[0]
42
Next, we get the index of the first review where VADER rated them as negative but TextBlob as neutral:
sent_scores[(sent_scores["vader_compound"] <= -0.8) & (sent_scores["textblob_polarity"].between(-0.1, 0.1))].index[0]
0
Let’s first retrieve the positive review:
sample_reviews[42]
"I love carpet sweepers for a fast clean up and a way to conserve energy. The Ewbank Multi-Sweep is a solid, well built appliance. However, if you have pets, you will find that it takes more time cleaning the sweeper than it does to actually sweep the room. The Ewbank does pick up pet hair most effectively but emptying it is a bit awkward. You need to take a rag to clean out both dirt trays and then you need a small tooth comb to pull the hair out of the brushes and the wheels. To do a proper cleaning takes quite a bit of time. My old Bissell is easier to clean when it comes to pet hair and it does a great job. If you do not have pets, I would recommend this product because it is definitely well made and for small cleanups, it would suffice. For those who complain about appliances being made of plastic, unfortunately, these days, that's the norm. It's not great and plastic definitely does not hold up but, sadly, product quality is no longer a priority in business."
This review seems mixed, but is overall somewhat positive.
Now, let’s look at the negative review:
sample_reviews[0]
'The only redeeming feature of this Cuisinart 4-cup coffee maker is the sleek black and silver design. After that, it rapidly goes downhill. It is frustratingly difficult to pour water from the carafe into the chamber unless it\'s done extremely slow and with accurate positioning. Even then, water still tends to dribble out and create a mess. The lid, itself, is VERY poorly designed with it\'s molded, round "grip" to supposedly remove the lid from the carafe. The only way I can remove it is to insert a sharp pointed object into one of the front pouring holes and pry it off! I\'ve also occasionally had a problem with the water not filtering down through the grounds, creating a coffee ground lake in the upper chamber and a mess below. I think the designer should go back to the drawing-board for this one.'
This review is unambiguously negative. From comparing the two, VADER appears more accurate, but it does tend to overly prioritize positive terms in a piece of text.
The final thing we can consider is how subjective versus objective each review is. We’ll do this by creating a histogram of TextBlob’s subjectivity score.
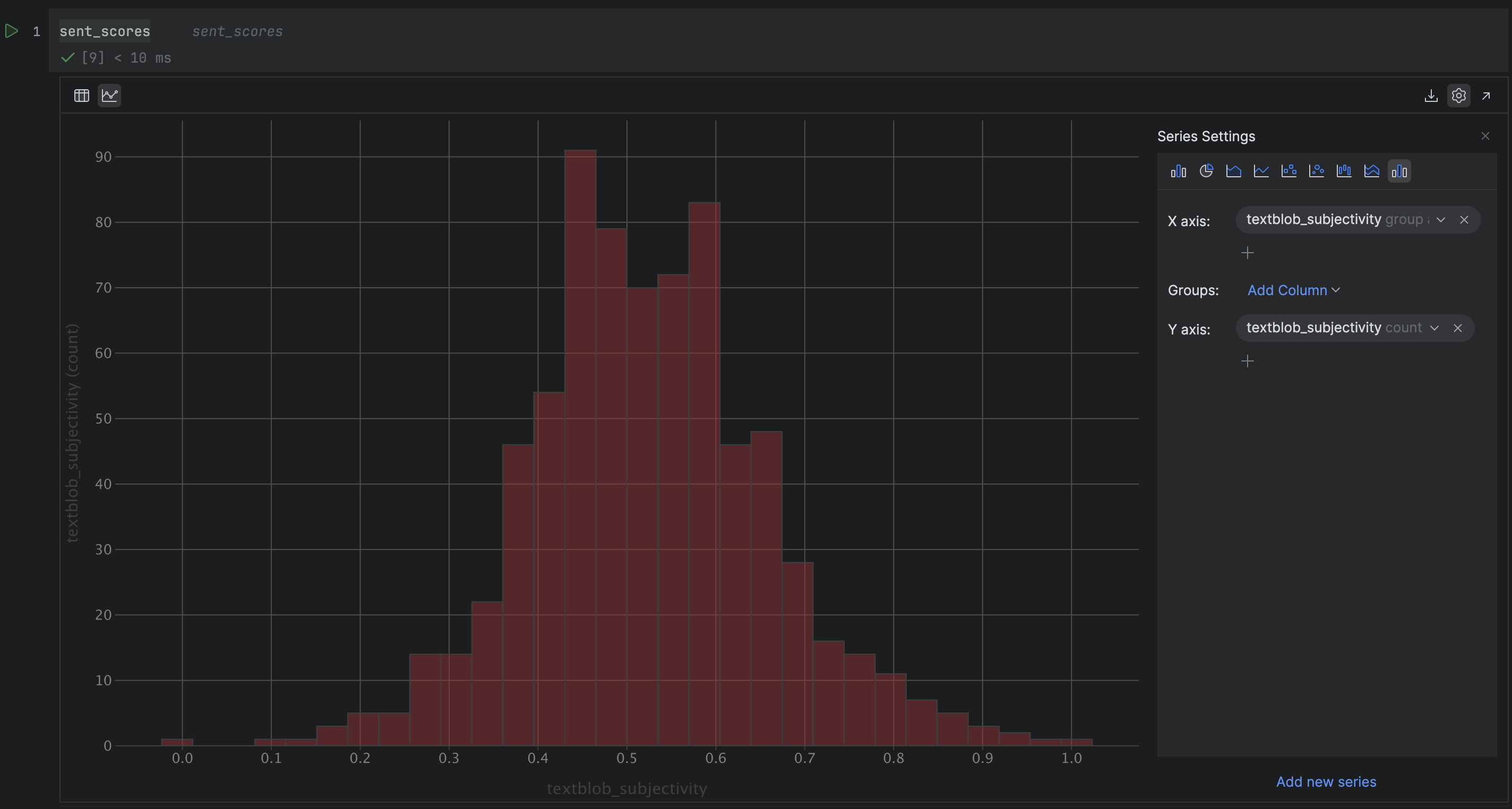
Interestingly, there is a good distribution of subjectivity in the reviews, with most reviews being a mixture of subjective and objective writing. A small number of reviews are also very subjective (close to 1) or very objective (close to 0).
These scores between them give us a nice way of cutting up the data. If you need to know the objective things that people did and did not like about the products, you could look at the reviews with a low subjectivity score and VADER compound scores close to 1 and –1, respectively.
In contrast, if you want to know what people’s emotional reaction to the products are, you could take those with a high subjectivity score and high and low VADER compound scores.
Things to consider
As with any problem in natural language processing, there are a number of things to watch out for when doing sentiment analysis.
One of the biggest considerations is the language of the texts you’re trying to analyze. Many of the lexicon-based methods only work for a limited number of languages, so if you’re working with languages not supported by these lexicons, you may need to take another approach, such as using a fine-tuned LLM or training your own model(s).
As texts increase in complexity, it can also be difficult for lexicon-based analyzers and bag-of-words-based models to correctly detect sentiment. Sarcasm or more subtle context indicators can be hard for simpler models to detect, and these models may not be able to accurately classify the sentiment of such texts. LLMs may be able to handle more complex texts, but you would need to experiment with different models.
Finally, when doing sentiment analysis, the same issues also come up as when dealing with any machine learning problem. Your models will only be as good as the training data you use. If you cannot get high-quality training and testing datasets suitable to your problem domain, you will not be able to correctly predict the sentiment of your target audience.
You should also make sure that your targets are appropriate for your business problem. It might seem attractive to build a model to know whether your products make your customers “sad”, “angry”, or “disgusted”, but if this doesn’t help you make a decision about how to improve your products, then it isn’t solving your problem.
Wrapping up
In this blog post, we dove deeply into the fascinating area of Python sentiment analysis and showed how this complex field is made more approachable by a range of powerful packages.
We covered the potential applications of sentiment analysis, different ways of assessing sentiment, and the main methods of extracting sentiment from a piece of text. We also saw some helpful features in PyCharm that make working with models and interpreting their results simpler and faster.
While the field of natural language processing is currently focused intently on large language models, the older techniques of using lexicon-based analyzers or traditional machine learning models, like Naive Bayes classifiers, still have their place in sentiment analysis. These techniques shine when analyzing simpler texts, or when speed, predictions, or ease of deployment are priorities. LLMs are best suited to more complex or nuanced texts.
Now that you’ve grasped the basics, you can learn how to do sentiment analysis with LLMs in our tutorial. The step-by-step guide helps you discover how to select the right model for your task, use it for sentiment analysis, and even fine-tune it yourself.
If you’d like to continue learning about natural language processing or machine learning more broadly after finishing this blog post, here are some resources:
- Learn how to do sentiment analysis with large language models
- Start studying machine learning with PyCharm
- Explore machine learning methods in software engineering
Get started with sentiment analysis in PyCharm today
If you’re now ready to get started on your own sentiment analysis project, you can activate your free three-month subscription to PyCharm. Click on the link below, and enter this promo code: PCSA24. You’ll then receive an activation code via email.