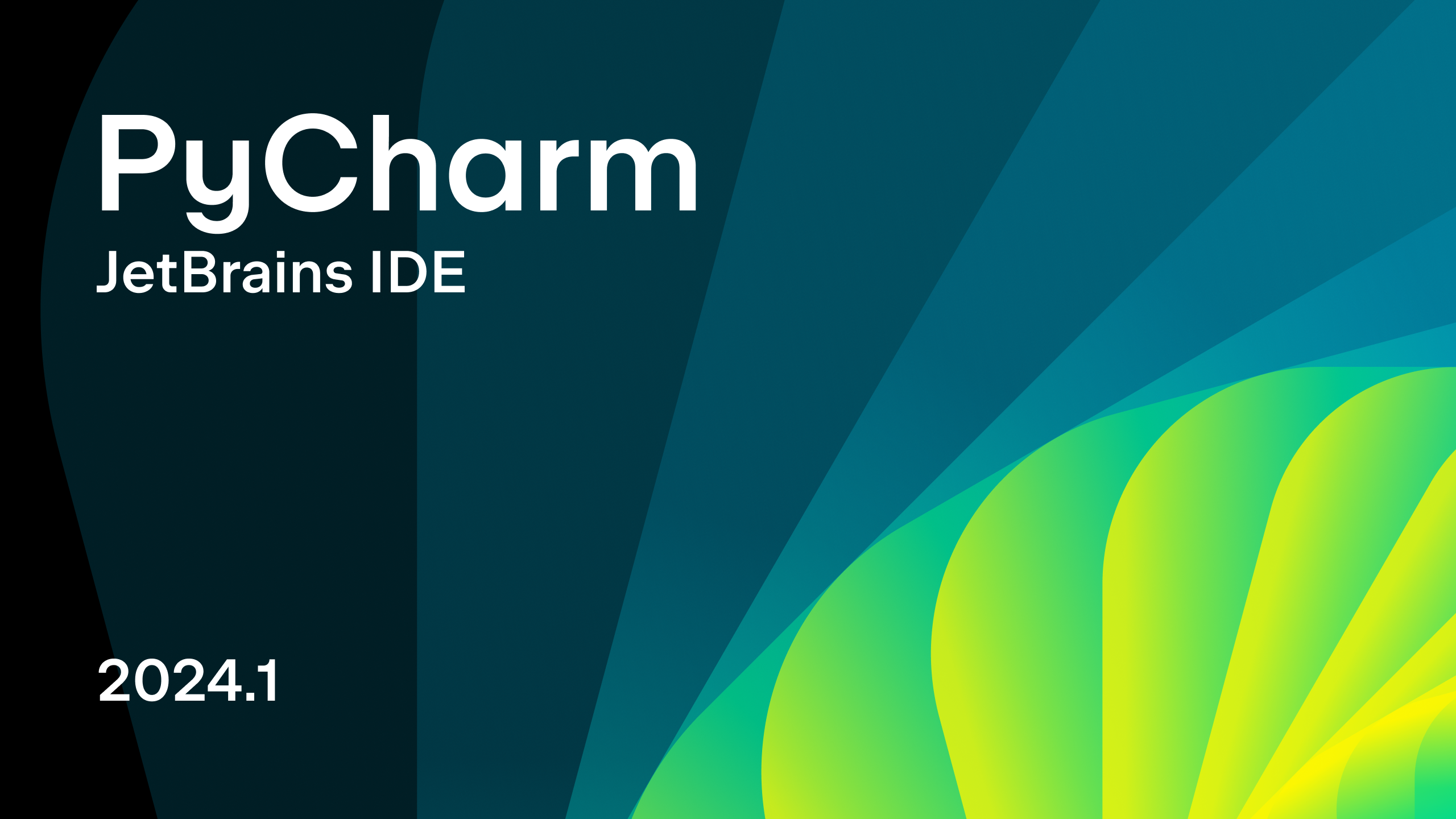
PyCharm 2024.1
PyCharm 2024.1 Is Here! Hugging Face Model Card Previews, Local Full Line Code Completion Updates, and more!
PyCharm 2024.1 is out with key updates, including full line code completion for frontend frameworks and documentation preview for Hugging Face models and datasets!